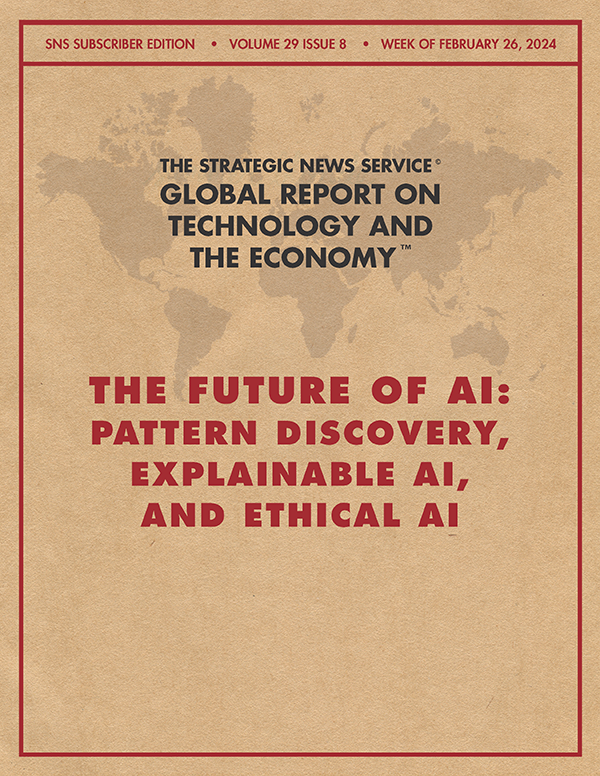
![]() |
THE FUTURE OF AI: PATTERN DISCOVERY, EXPLAINABLE AI, AND ETHICAL AI By Mark Anderson
Why Read: At a time when the world has gone gaga over a piece of software that does little more than reflect our language, it is worth asking what the next 10 years of AI might look like, long after the love affair - and pendular blowback - are over. To move beyond the "fancy mirror" of GPT, keep reading. _____ It takes a long time for new AI platforms to mature, and perhaps an equally long time for us to move beyond them. Neural nets began in the 1960s - or, some say, earlier. And even generative AI and large language models (LLMs) morphed from smaller neural nets (NNs), adding transformers, reinforcement learning, etc.; but they've been around for many years, invented by Google and somehow ending up at Microsoft. And while the ChatGPT adoption rate, with the advent of massive scaling of training sets (less a brilliant move than an expensive one), has been record-setting, this technology is - today, at least - not trustworthy. Why OpenAI was willing to ship this product, marketed as a chat bot, when many others were not may be something the company and other users will be answering for, for many years to come. One very good way to see where a technology is going in the future is to imagine already being there and looking back. When we look back from 2034, we will see the cost of shipping flawed generative pre-trained transformer (GPT) software to every business and government, and we will marvel that so many would be so excited over an untrustworthy advanced natural-language processor. We will understand that our excitement was as emotional as it was technical, hyped on by false claims of inevitable artificial general intelligence (AGI), when it turned out the opposite was the case: LLMs were never going to lead to AGI. In short, there is a difference between the "fancy mirror" role of LLMs vs. a path to new scientific discoveries. To be clear, one expects that LLMs will continue their massive adoption curve and be important - particularly in fields and uses in which the cost of risk or failure is very, very low. It is likely, over this period, that whole new forms of visual art, video, music, and poetry are created and become mainstream, enjoyed by millions or even billions, while performing advisory roles in requiring human review elsewhere. From this new perspective, we see the early shoots, back in 2024, of the platforms and technologies that would become the AI tools of the future, providing trusted outcomes, explainable and interpretable (as Eric Schmidt and Elon Musk have properly prescribed), and capable of making major new scientific discoveries - none of which is true of the LLMs of today. While it is beyond the scope of this piece to do a deep dive into the technologies and mathematics that likely will prevail in 10 years, there is a more useful approach for such exploration: predicting the necessary functions, in logical and chronological order, that we should expect to see and use. |