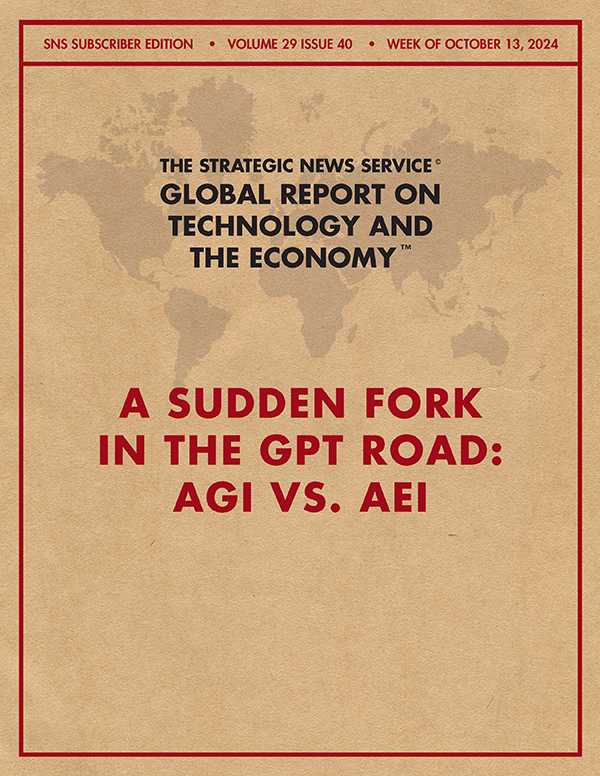
![]() |
A SUDDEN FORK IN THE GPT ROAD: AGI vs. AEI By Mark Anderson Why Read: While most of the tech world is busy wrestling with what to do with a product type (large language models, or LLMs) that is opaque in its workings and untrustworthy in its outputs, there is a different fork in the app path of this kit that is both more interesting and worrying. As unlikely as it is that LLMs will ever fulfill the hype about enterprise-wide use and problem solving, there is every reason to believe that they will excel in engaging, and mirroring, individual users. After all, what do we like more than seeing ourselves in the mirror? In this week's discussion, we'll take a close look at the question of whether the real destiny of LLMs is to engage with individual humans - to help, to learn, and to manipulate. And the greatest risk here? We will welcome it. - mra __________ When there's a fork in the road, take it. - Yogi Berra You don't have to run faster than the bear to get away. You just have to run faster than the guy next to you. - Jim Butcher (?)
It appears that there are two separate but related trends emerging in the generative AI space: an increased sense of the role of generative pretrained transformers (GPTs) in producing music, film, art, movies, and media content; and a growing list of trust-dependent enterprise jobs one would (in Sam Altman's words) "never" use GPT to do. An important fork in the future technology roadmap is suddenly becoming apparent. The first is led by the OpenAI axis; we all know it as the search for artificial general intelligence (AGI). The second, originally led (as well as resisted) by artists, writers, and the creative world, has a different goal entirely, just as arts and business have different goals. This forked path in AI applications could be described as the difference between the original IBM Watson (a fast reader but not much else) and Freud as seen through our language. More important, this forked path represents the difference between a large language model (LLM) making great new discoveries in, say, math and physics versus an LLM getting better at predicting human attributes based on human language use. Can you guess which one works better? |